AI tools are more widely used than ever before. Many organisations are examining the benefits AI can deliver and the UK Government is looking for us to be an AI-enabled country. What challenges does AI present from a data protection (DP) perspective and how do these challenges affect the approach we take to achieving compliance?
From following instructions to self-learning. What is artificial intelligence?
There isn’t an agreed legal definition of ‘artificial intelligence’, not least because it can cover a wide scope of technology. However, it typically includes those systems that learn from datasets rather than simply following a list of pre-programmed rules.
Most implementations of AI involve a two-stage process. The first stage is a training, development or learning phase, whereby the system looks at a dataset (often a very large dataset) to determine its characteristics. The second is a production or deployment stage, where the system is asked to produce outcomes from the learning it has made in the first stage. Both stages serve different purposes, both can process personal data and both present different data protection challenges.
But as the reactions are learned and not programmed, how that system has reached a conclusion may not be entirely clear; it operates in what is often called a ‘black box’. This underlying characteristic produces inherent difficulties with achieving DP compliance which do not occur in traditional personal data processing. This can include opacity about the source of the original learning data, bias introduced through reliance on the original data or through algorithms, and inadvertent use of personal data or intellectual property.
In this blog, we will start by looking at how the guidance and regulation is evolving. We will then go on to examine aspects of DP compliance, how AI poses new challenges to old requirements, and then summarise the issues in a list of aspects to consider.
From technological innovation and government aspirations to effective regulation. How is the regulatory framework evolving?
The UK government published a white paper in March 2023 entitled ‘A pro-innovation approach to AI regulation’, which focussed on adopting and adapting existing legislation and regulatory regimes instead of the European Union’s (EU’s) more prescriptive and legislation-driven approach taken in its EU AI Act, recently adopted by MEPs.
In August 2023, a House of Commons' report suggested that the White Paper's approach risked the Government being left behind by the EU’s more prescriptive tactic and proposed an AI Bill to be included in the King’s Speech on 7 November 2023, although that recommendation was not taken up.
The Government’s intention seems to be to provide oversight via the Information Commissioner’s Office (ICO) and other existing regulatory bodies including Ofcom and the Financial Conduct Authority. (It is worth noting at this stage that the ICO’s role might be reformed by the Data Protection and Digital Information Bil No.2 or ‘DPDI’ currently going through parliament.)
The ICO-issued guidance on how to use AI and personal data in late 2022, around the time that it collaborated with the Alan Turing Institute to issue a paper on explaining decisions made with AI. Updated ICO guidance on AI was issued in March 2023.
New problems for established requirements. What are the key aspects of data protection that AI causes us to consider in a different way?
Some of the established AI services, such as ChatGPT, Bing AI or LaMDA, use large language models to generate text in response to an input from a series of questions or instructions. The service then provides answers drawing on its learning from the large language model through a process of recognising, predicting and generating content. One of the initial risks is that personal data may be included in that large language model which will find its way into the generated output. The mainstream AI services are infamously vague about the origins of the data sources, so users of the output may never realise that their output contains, or was generated from, personal data or information forming the intellectual property of others.
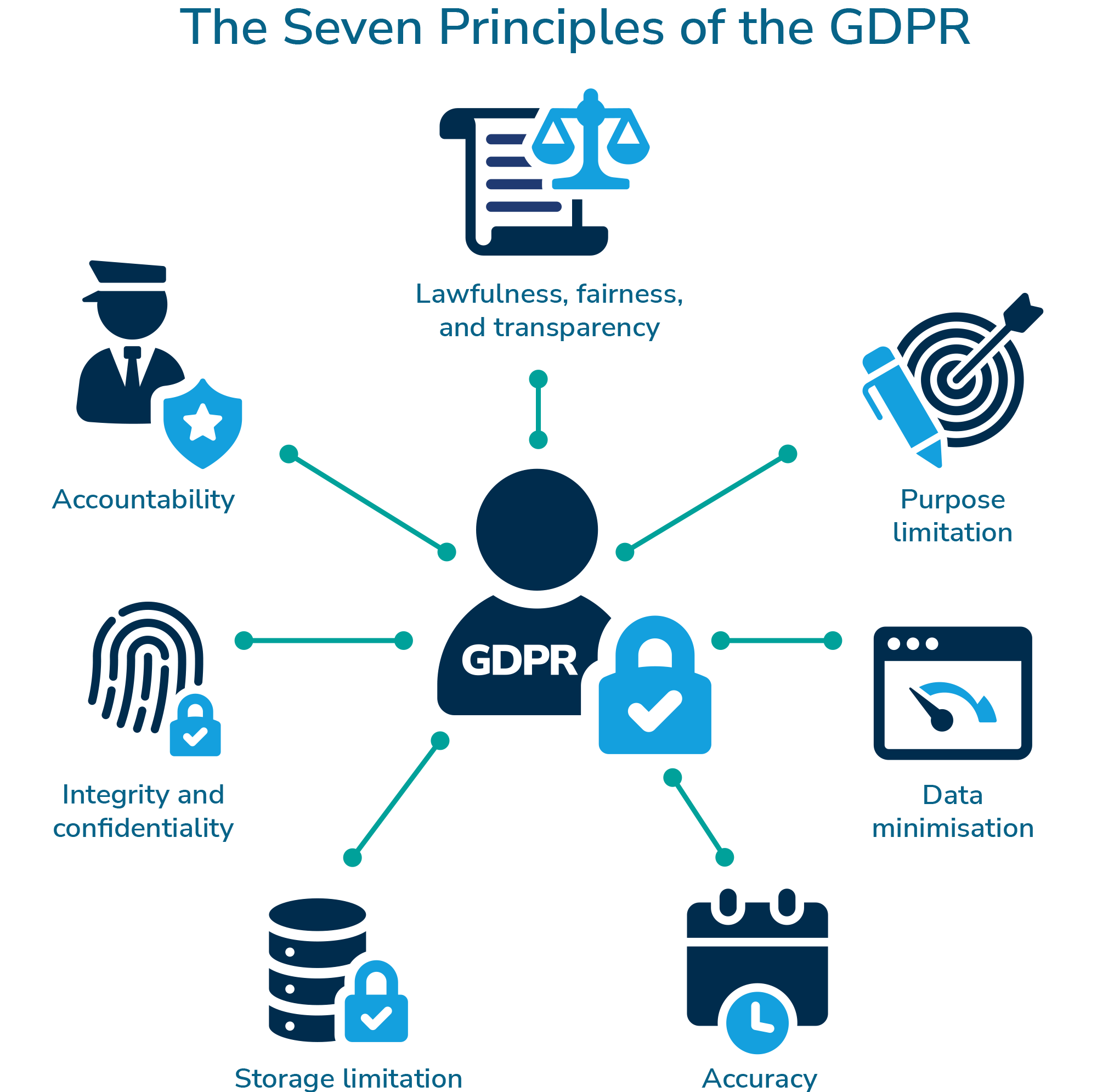
The principles of the UK General Data Protection Regulation (UK GDPR) include lawfulness, fairness, transparency, purpose limitation, data minimisation, accuracy, storage limitation and rules in relation to automated decision-making. Let’s take a look at some of them in a bit more detail.
Fairness and lawfulness
To process personal data, you must have an appropriate lawful basis. As the use of AI involves two processing stages, i.e., the training and production stages, this means ensuring that there's a lawful basis for each type of processing and the lawful basis may be different for each stage.
If the proposal involves processing special category data (e.g., health, race, religious beliefs, politics, biometrics, sex life or sexual orientation, etc.), then there is a need to identify an additional legal basis under Article 9. One potential Article 9 basis is explicit consent, but this is often difficult to acquire in practice, particularly given the challenges of explaining how AI would process an individual’s personal data (more of this later). However, this may be the only option if, for example, there is no ‘substantial public interest’ such as the prevention or detection of crime. Furthermore, there are likely to be considerations of necessity and proportionality – context, after all, is everything.
As understanding AI is impenetrable to many, ensuring that processing is fair can be a challenge, not least because fairness centres around processing data only in a manner which individuals would reasonably expect. It remains an issue that AI systems can reflect bias in the learning datasets or create bias where none previously existed. It can perpetuate bias that stems from the learning data or programming algorithms, therefore it is important to ensure that the system is sufficiently statistically accurate and thoroughly tested for bias before implementation.
Transparency
The UK GDPR requires a controller to inform data subjects what information they hold about them and how they are using it. The complexity of understanding how AI systems work and how they might process personal data translates into a perceived lack of transparency, which can make it difficult for controllers to explain those decisions to data subjects if the algorithm and data sources are opaque. The ICO has recognised the difficulty of ‘explainability’ and has produced guidance in collaboration with the Alan Turing Institute, ‘Explaining decisions made with AI’. The EU’s Article 29 Working Party in its ‘Guidelines on automated individual decision making and profiling WP251’ said ‘The process of profiling is often invisible to the data subject. It works by creating derived or inferred data about individuals – ‘new’ personal data that has not been provided directly by the data subjects themselves. Individuals have differing levels of comprehension and may find it challenging to understand the complex techniques involved in profiling and automated decision-making processes.’
In certain circumstances the right to be informed can be waived if the data was not collected from the data subject, for example, if it can be demonstrated that informing data subjects is impossible or requires disproportionate effort (UK GDPR Article 14). However, for the exercise of rights, such as the Article 22 right relating to automated decision-making, it is essential to provide detailed explanations to the data subject. Even if regulators recognise the difficulty in explaining AI processing, there is, nonetheless, an obligation for controllers to provide as full a description as possible about the source of the information and how any outcomes have been derived, in the plainest possible language.
Purpose limitation
The purpose limitation principle requires that personal data must only be collected for specified, explicit and legitimate purposes and not processed further. Learning data for AI can be collected through a process called data scraping or web scraping, where data from various sources is compiled on a huge scale and may include sources from social media and the internet in general. Due to the almost indiscriminate nature of this process and its sheer scale, personal data will be included without any record that it has been included, and it may also collect data which is untrue or inaccurate, or which forms the intellectual property of others. Consequently, this method is almost the exact opposite of that required to comply with the purpose limitation principle.
Data minimisation
The UK GDPR requires the processing of personal data to be adequate, relevant and limited to what is necessary. The nature of compiling huge datasets for learning models means that, again, this process is at the opposite end of the scale from only processing what is necessary and no more. This is particularly troublesome where the dataset includes special category data.
Accuracy
Any personal data needs to be accurate and, where necessary, kept up to date. As data is collected in such large volumes for learning models, it is not always possible for it to be verified or checked in any way. Moreover, the data, once in the model, may be unrecognisable and very difficult to correct. Inaccurate information, personal data or otherwise, and information which introduces or produces bias, is one of the main issues with commercial AI chatbot services.
Storage limitation
Personal data must be kept in a form which allows identification of data subjects for no longer than is necessary. Again, as with the accuracy principle, this is difficult to achieve with many AI models. However, provided a controller can justify longer retention periods for data in the learning systems which is proportionate to the purpose of the AI system, there is no reason why data couldn’t be kept longer than would be the case in a conventional scenario.
Accountability
There is a need to maintain good records from the beginning of the development, which will not only enable a controller to demonstrate GDPR compliance but will help to explain the processing to data subjects and regulators.
Automated decision-making
If an AI model is intended to make automated decisions about individuals, further challenges are presented. As the law currently stands, data subjects have a right not to have decisions made about them purely by automated means on the basis that important decisions, such as for a mortgage or a job application, should not be handled solely by an algorithm. The first hurdle is that you must tell data subjects about the automated decision-making and how it works, which brings us back to the issues of transparency and ‘explainability’ inherent in such a complex process.
One solution is human intervention – requiring a human to ratify the decision made by the model. But with the complexity of AI systems and their data sources, would it be possible for a human to make an informed and meaningful assessment about whether the model’s decision was correct? An alternative solution is to undertake the automated decision-making with the data subject’s consent, but this again involves explaining the decision-making process so that the consent is ‘fully informed’.
The current DPDI Bill going through parliament contains proposals to make changes to the rules on automated decision-making, whereby it would be lawful to make automated decisions in some pre-defined circumstances. Whilst those circumstances are limited in the Bill, it also gives the power to the Secretary of State to extend that list or circumstances, which may open the door to purely automated AI decision-making for some purposes.
Data subject rights
AI services present problems with the practicalities of meeting data subjects’ rights requests, such as the rights of access, rectification, erasure and so on, because personal data in AI models is often difficult to identify and extract. Also, the rights apply to both the personal data contained in the learning model and those contained in the output of production. To avoid arriving at a place where data subject rights cannot be met, the principle of data protection by design and default should be adopted, with the exercise of rights built into the solution at the earliest stage.
New problems need new solutions. What could be considered to achieve data protection compliance in AI systems?
One of the most significant risks associated with AI is the speed of change, as data controllers rush to deploy the benefits of AI. Many controllers are outsourcing the development of AI systems to specialist third-party developers, not always with properly developed detailed system specifications, which results in only the developers understanding how they work. Contracts that ensure lasting ownership of specifications, intellectual property, patents and support could become critical as small software firms are acquired and absorbed into bigger firms as the industry progresses.
Implementing a continuous improvement approach
There are AI systems which learn continuously, where data used or produced in production is fed back into the learning model to improve learning and effectiveness. There are risks that some of the key characteristics will creep, introducing or worsening discriminatory bias and the deterioration of the accuracy of output. This arises from the use of the same data for two purposes – that for which the system is intended to use in production, and the intrinsic improvement of the learning model.
This requires a continuous monitoring and assessment regime which can detect issues early and identify improvements. (The assessment of AI systems is a key part of the EU’s AI legislation.) It is necessary for validating the learning phase with the aim of verifying objectively that the learning performs in the way it was designed and is suitable for deployment into the production phase. It also minimises the risks of scope creep over time, particularly if the profiles of the individuals whose personal data make up the learning data is different from those at which it is aimed in production. It also ensures that the system in production meets the operational requirements for which it was designed, because the quality of the learning data is no guarantee of good quality in production outputs.
Avoiding discrimination and minimising data
There is a need to determine the data necessary to train the system and not to rely on a ‘more is better’ approach. The quality of the learning data must be balanced against the purpose of the system in production and in line with the proportionality principle. Some things to think about when building a learning dataset are:
- Deciding whether personal data is necessary for the model, whether it can be anonymised or pseudonymised, or whether filtering or masking mechanisms can be used
- Distinguishing between data for the learning and production phases
- Documenting the process in line with the accountability principle, including sources, data compilation and verification of data integrity
- Regularly reassessing for privacy risks of data subjects in line with their rights
- Being vigilant with information security.
Consider appropriate risk mitigations
Despite the new and unique data protection challenges posed by AI, it does not necessarily mean that deployment of compliant AI systems is not possible, but it does mean that those new risks should be mitigated appropriately.
Those mitigations might include:
- Thoroughly testing the system on a reliable dataset with outcomes analysed to ensure they are commensurate with the objectives and the law. In the rush to completion, developers need to be certain that learning datasets have been established from lawfully obtained data processing.
- Sampling outputs from the system to be reviewed on an ongoing basis to confirm the quality of outcomes, particularly where outcomes are fed back into the learning dataset.
- Adding confidence limits on outcomes, with associated flags for any outcomes which exceed pre-determined limits.
- Providing quality responses in reaction to automated decisions which explain the process and why the decision has been made. For example, if a mortgage application is rejected, the feedback could be that the application would have been accepted had the loan amount been lower by X or if the applicant’s income had been higher by Y.
- Building in the ability for human understanding and intervention in outcomes, and training those analysing outputs to understand how the model derives them.
- Learning from others - for example, there are controls required for algorithmic trading by financial services firms in the Markets in Financial Instruments Directive (MiFID II).
Produce an explanation for how the system works
At the earliest stage, a description of how the system works needs to be written in the plainest possible language, including the source of the learning data, its accuracy or output and the mitigations in place to avoid bias, error and discrimination. Focus groups can also be used to test out the explanation, so that it can be used with confidence when presenting to employees, data subjects and the regulator.
Use established methods to assess and record your decisions
Data protection impact assessments (DPIAs) are mandatory for high-risk processing and the process will be familiar to data protection officers (DPOs). DPIAs provide an essential template to record the risks, mitigations and design thought processes. If the lawful basis is legitimate interests, then a legitimate interests assessment (LIA) is essential. It comprises a purpose test to identify the legitimate interest of the processing, a necessity test to consider if that processing is necessary and a balancing test against the data subjects’ interests.
How URM can help?
Consultancy
With nearly 2 decades of experience providing data protection and GDPR consultancy, URM is ideally placed to help your organisation maintain compliance with DP legislation as it leverages newly-developed technologies, such as AI. Our large team of GDPR consultants can support your organisation’s efforts to remain compliant in its deployment of AI systems, making sure to always remain completely up to date with the latest guidance in this rapidly evolving regulatory landscape.
The GDPR consultancy services we offer range from conducting a gap analysis of your current processing practices against regulatory requirements and providing remediation support, through to providing assistance with the completion of specific compliance activities, such as DPIAs and records of processing activities (RoPAs). If your organisation receives data subject access requests (DSARs), we can help you compliantly respond to these by providing a GDPR DSAR redaction service, where we will help you identify any applicable exemptions and remove information that cannot be disclosed to the data subject. We can also provide ongoing support in the form of a virtual DPO service, which enables you to access an entire team of highly qualified and experienced DP practitioners.
Training courses
Alongside providing compliance support in the form of consultancy, URM can also help you enhance your own understanding of your DP compliance obligations by offering a range of DP-related training courses, all of which are led by a practising GDPR consultant. We regularly run courses on how to conduct a DPIA, a data transfer impact assessment (DTIA), and how to respond to a DSAR request in full compliance with the Regulation. Meanwhile, if you would like to gain an industry-recognised DP qualification, we regularly deliver the BCS Foundation Certificate in Data Protection (CDP) course.
URM can offer a host of consultancy services to improve your DP policies, privacy notices, DPIAs, ROPAs, data retention schedules and training programmes etc.
By attending URM’s online BCS Foundation Certificate in Data Protection course, you will gain valuable insights into the key aspects of current DP legislation including rights of data subjects and data controller obligations.
If uncertain, URM is able to conduct a high-level GDPR gap analysis which will assist you understand your current levels of compliance and identify gaps and vulnerabilities.

We have seen an increased focus on the General Data Protection Regulation (GDPR) by certification body (CB) assessors when conducting ISO 27001 audits.

URM’s blog outlines the DP concerns around the use of facial recognition technology (FRT), and offers guidance on making sure your FRT use is GDPR compliant.

BS 10012 is a standard which has been developed to enable organisations to implement a personal information management system (PIMS).